[工程] gunicorn下的深度學習api 如何合理分配gpu
背景
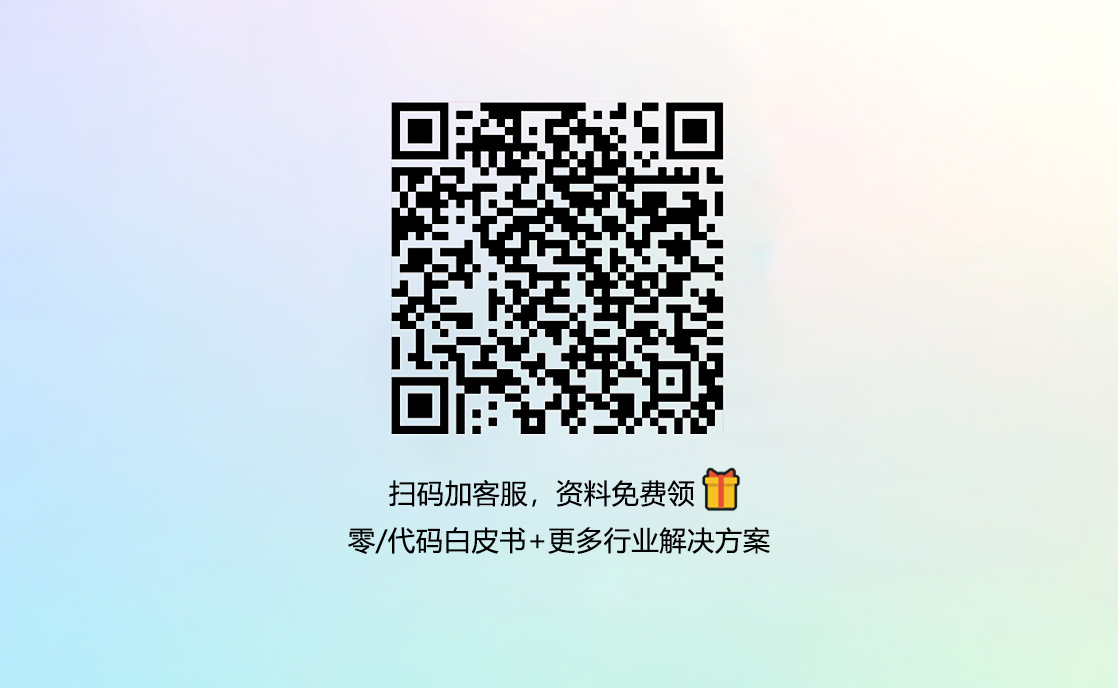
老大提了一個需求: gunicron 起多個進程的時候,如何保證pytorch的模型均勻分配到不同的gpu上,按道理,如果能拿到類似每個進程的序號,那分配起來應該都是簡單的,那核心問題提煉出來了,如何拿到進程的序號
分析
順手直接去找一個相關的問題和分析,https://github.com/benoitc/gunicorn/issues/1278 ,發現很多人都有同樣的需求,不過貌似提的pr都沒有進一步的解決,所以只能進一步來看官方的文檔有什么可用的。
通過進一步發現 http://docs.gunicorn.org/en/latest/settings.html 的文檔,這些在起進程的時候就可以預先定義好進程的id
實踐
我們寫好gunicorn_conf.py
# RTFM -> http://docs.gunicorn.org/en/latest/settings.html#settings import os from service.config import WORKERS bind = '0.0.0.0:2048' workers = WORKERS timeout = 300 max_requests = 2000 max_requests_jitter = 500 def on_starting(server): """ Attach a set of IDs that can be temporarily re-used. Used on reloads when each worker exists twice. """ server._worker_id_overload = set() def nworkers_changed(server, new_value, old_value): """ Gets called on startup too. Set the current number of workers. Required if we raise the worker count temporarily using TTIN because server.cfg.workers won't be updated and if one of those workers dies, we wouldn't know the ids go that far. """ server._worker_id_current_workers = new_value def _next_worker_id(server): """ If there are IDs open for re-use, take one. Else look for a free one. """ if server._worker_id_overload: return server._worker_id_overload.pop() in_use = set(w._worker_id for w in server.WORKERS.values() if w.alive) free = set(range(1, server._worker_id_current_workers + 1)) - in_use return free.pop() def on_reload(server): """ Add a full set of ids into overload so it can be re-used once. """ server._worker_id_overload = set(range(1, server.cfg.workers + 1)) def pre_fork(server, worker): """ Attach the next free worker_id before forking off. """ worker._worker_id = _next_worker_id(server) def post_fork(server, worker): """ Put the worker_id into an env variable for further use within the app. """ os.environ["APP_WORKER_ID"] = str(worker._worker_id)
1
2
3
4
5
6
7
8
9
10
11
12
13
14
15
16
17
18
19
20
21
22
23
24
25
26
27
28
29
30
31
32
33
34
35
36
37
38
39
40
41
42
43
44
45
46
47
48
49
50
51
52
53
54
55
56
57
58
59
60
61
62
63
64
# RTFM -> http://docs.gunicorn.org/en/latest/settings.html#settings
import os
from service.config import WORKERS
bind = '0.0.0.0:2048'
workers = WORKERS
timeout = 300
max_requests = 2000
max_requests_jitter = 500
def on_starting(server):
"""
Attach a set of IDs that can be temporarily re-used.
Used on reloads when each worker exists twice.
"""
server._worker_id_overload = set()
def nworkers_changed(server, new_value, old_value):
"""
Gets called on startup too.
Set the current number of workers.??Required if we raise the worker count
temporarily using TTIN because server.cfg.workers won't be updated and if
one of those workers dies, we wouldn't know the ids go that far.
"""
server._worker_id_current_workers = new_value
def _next_worker_id(server):
"""
If there are IDs open for re-use, take one.??Else look for a free one.
"""
if server._worker_id_overload:
return server._worker_id_overload.pop()
in_use = set(w._worker_id for w in server.WORKERS.values() if w.alive)
free = set(range(1, server._worker_id_current_workers + 1)) - in_use
return free.pop()
def on_reload(server):
"""
Add a full set of ids into overload so it can be re-used once.
"""
server._worker_id_overload = set(range(1, server.cfg.workers + 1))
def pre_fork(server, worker):
"""
Attach the next free worker_id before forking off.
"""
worker._worker_id = _next_worker_id(server)
def post_fork(server, worker):
"""
Put the worker_id into an env variable for further use within the app.
"""
os.environ["APP_WORKER_ID"] = str(worker._worker_id)
這樣我們通過環境變量就可以清楚的知道我們的當前子進程的序號
# -*- coding: utf-8 -*- import os import torch def set_process_gpu(): worker_id = int(os.environ.get('APP_WORKER_ID', 1)) devices = os.environ.get('CUDA_VISIBLE_DEVICES', '') if not devices: print('current environment did not get CUDA_VISIBLE_DEVICES env ,so use the default') rand_max = 9527 gpu_index = (worker_id + rand_max) % torch.cuda.device_count() print('current worker id {} set the gpu id :{}'.format(worker_id, gpu_index)) torch.cuda.set_device(int(gpu_index))
1
2
3
4
5
6
7
8
9
10
11
12
13
14
15
16
17
18
19
# -*- coding: utf-8 -*-
import os
import torch
def set_process_gpu():
worker_id = int(os.environ.get('APP_WORKER_ID', 1))
devices = os.environ.get('CUDA_VISIBLE_DEVICES', '')
if not devices:
print('current environment did not get CUDA_VISIBLE_DEVICES env ,so use the default')
rand_max = 9527
gpu_index = (worker_id + rand_max) % torch.cuda.device_count()
print('current worker id??{} set the gpu id :{}'.format(worker_id, gpu_index))
torch.cuda.set_device(int(gpu_index))
通過這個方法就可以輕松的設置自己進程所在的gpu ,這樣就可以根據gpu的數量,均勻的分配進程
gunicorn -c gunicorn_conf.py wsgi:app
1
2
gunicorn -c gunicorn_conf.py wsgi:app
wsgi.py 這個就是app的實體了,正常啟用就可以了。
API GPU加速云服務器 深度學習
版權聲明:本文內容由網絡用戶投稿,版權歸原作者所有,本站不擁有其著作權,亦不承擔相應法律責任。如果您發現本站中有涉嫌抄襲或描述失實的內容,請聯系我們jiasou666@gmail.com 處理,核實后本網站將在24小時內刪除侵權內容。
版權聲明:本文內容由網絡用戶投稿,版權歸原作者所有,本站不擁有其著作權,亦不承擔相應法律責任。如果您發現本站中有涉嫌抄襲或描述失實的內容,請聯系我們jiasou666@gmail.com 處理,核實后本網站將在24小時內刪除侵權內容。